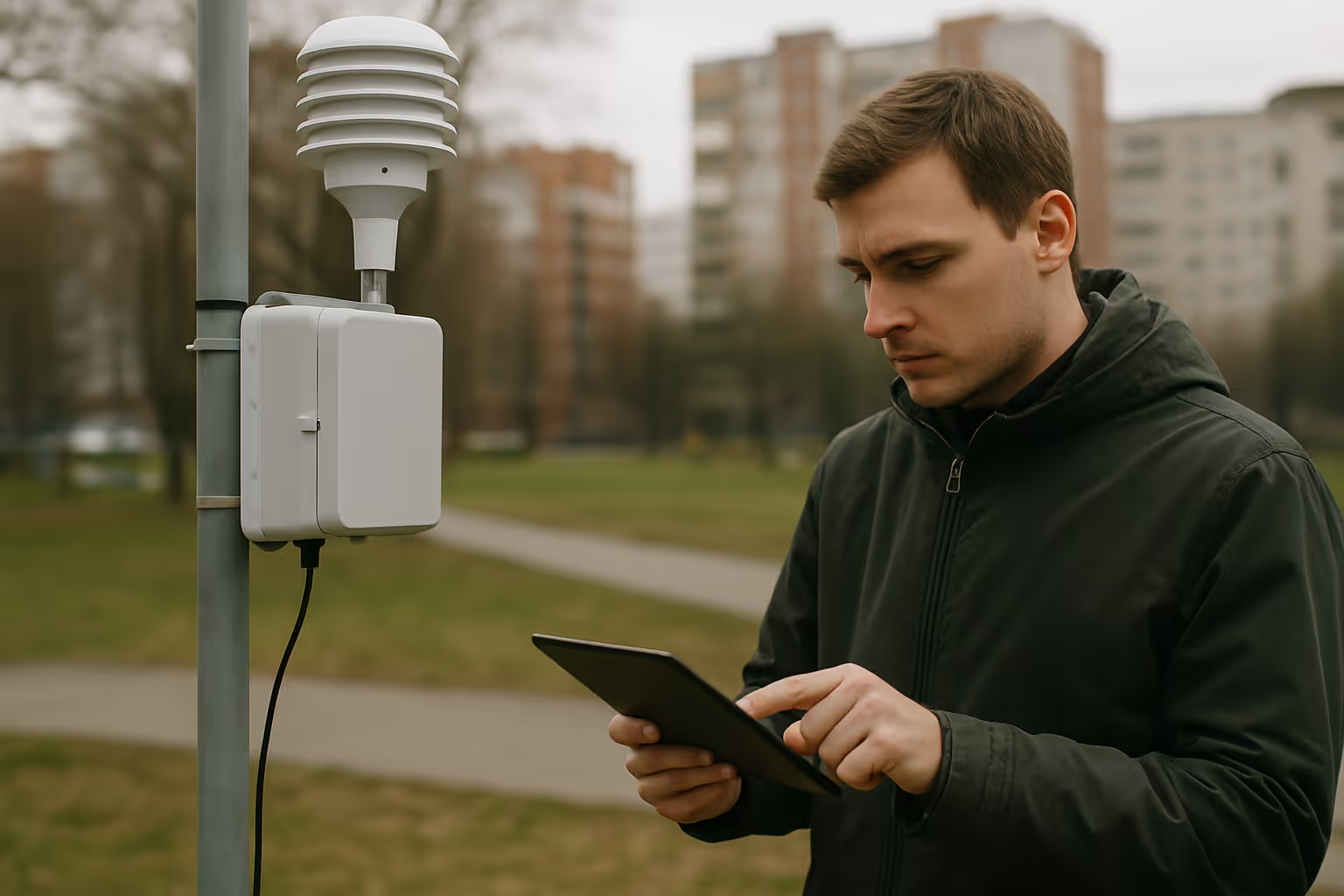
The Power of Hyperlocal Monitoring: Deploying IoT Sensor Networks for Granular Air Quality Data
Urban air quality is a critical determinant of public health and environmental sustainability. While the importance of monitoring air pollution has long been recognized, traditional methods often provide a broad, city-wide average that can mask significant variations in air quality at the neighborhood or even street level. These "monitoring deserts" mean that both citizens and policymakers lack the detailed information needed to understand the air they are truly breathing and to implement effectively targeted interventions. The rise of smart city technologies, particularly the Internet of Things (IoT), is changing this paradigm by enabling hyperlocal air quality monitoring – the collection of granular, real-time data at a much finer spatial and temporal resolution. This capability is unlocking new insights into urban air pollution dynamics and empowering cities to take more precise and impactful action towards cleaner air.
The Limitations of Traditional Air Quality Monitoring
For many years, urban air quality monitoring has primarily relied on a limited number of large, complex, and expensive regulatory-grade monitoring stations. While these stations provide highly accurate and reliable data that is essential for compliance reporting and long-term trend analysis, their cost and the infrastructure required for their operation mean they are typically few and far between within a city. This results in large gaps in monitoring coverage, where air quality can vary significantly from the nearest monitoring station due to localized sources like busy intersections, industrial facilities, or even construction sites. Consequently, city-wide average data doesn't reflect the actual exposure levels experienced by residents in different microenvironments, making it difficult to identify specific problem areas or assess the true impact of localized emissions.
Understanding Hyperlocal Air Quality Monitoring
Hyperlocal air quality monitoring addresses the limitations of traditional networks by deploying a much denser network of sensors. This approach focuses on capturing air quality data at a highly localized level – perhaps every few blocks or even on individual streets – and updating this data frequently, often in near real-time. This level of granularity reveals the intricate spatial and temporal variations in urban air pollution that are invisible to sparser networks. It allows for the identification of specific pollution hotspots, helps to pinpoint contributing sources with greater accuracy, and provides a more realistic understanding of the air quality conditions in the diverse microenvironments where urban residents live, work, and travel.
The Foundation: IoT Sensor Networks for Granular Data Collection
The feasibility and scalability of hyperlocal air quality monitoring are fundamentally enabled by the Internet of Things (IoT). IoT technology allows for the interconnection of a vast number of devices, in this case, air quality sensors, enabling them to collect and transmit data wirelessly to a central platform. The development of smaller, more affordable, and lower-power air quality sensors, often referred to as low-cost sensors (LCS), is a key driver behind the proliferation of these dense networks. While LCS may have different performance characteristics compared to regulatory monitors, their significantly lower cost per unit allows for their deployment in much larger numbers, providing the necessary spatial density for hyperlocal coverage.
Key Technologies Enabling Hyperlocal Air Quality Monitoring
A combination of interconnected technologies forms the backbone of effective hyperlocal air quality monitoring systems:
- Low-Cost Air Quality Sensors: These are the primary data collection units in a hyperlocal network. They utilize various sensing technologies (e.g., electrochemical, metal oxide semiconductor, optical particle counters) to measure concentrations of key pollutants such as PM2.5, PM10, NO2, O3, CO, and SO2. While more susceptible to environmental factors like temperature and humidity, ongoing advancements are improving their accuracy and reliability. The advantage lies in their affordability, enabling widespread deployment.
- IoT Connectivity and Communication Protocols: Transmitting data from potentially hundreds or thousands of sensors across a city requires robust and efficient wireless communication. Low Power Wide Area Network (LPWAN) technologies like LoRaWAN and NB-IoT are particularly well-suited for this purpose due to their ability to transmit small amounts of data over long distances with minimal power consumption, extending sensor battery life. Other options include standard cellular networks (3G, 4G) and Wi-Fi, depending on the specific deployment context and data transmission needs.
- Edge Computing for Local Data Processing: In dense networks, processing all raw data in the cloud can be inefficient. Edge computing involves performing some initial data processing, filtering, or aggregation at the sensor level or on local gateways before transmitting the data to the cloud. This reduces the volume of data sent over the network, decreases latency, and can enable faster local alerts or responses.
- Cloud Platforms for Data Ingestion and Storage: The massive volume of granular data generated by a hyperlocal network necessitates a scalable and robust cloud-based platform for data ingestion, storage, and management. Cloud infrastructure provides the processing power and storage capacity required to handle continuous streams of data from numerous sensors.
- Data Calibration, Validation, and Quality Control: A critical aspect of using data from low-cost sensors is ensuring its accuracy and reliability. This requires rigorous calibration and validation processes. Calibration often involves co-locating LCS with regulatory-grade monitors to develop correction algorithms that account for biases caused by environmental factors or sensor drift. Ongoing data validation and quality control procedures are essential to maintain data integrity over time.
- Data Analytics and Visualization Tools: Once collected and validated, the granular data needs to be processed, analyzed, and presented in an understandable format. Advanced analytics, including AI and machine learning, are used to identify patterns, correlate data from different sensors and sources, identify pollution sources, and predict future air quality trends. Visualization tools, such as high-resolution pollution maps, dashboards, and real-time graphs, are crucial for making the complex data accessible and actionable for different stakeholders.
Strategies for Deploying and Managing Hyperlocal Networks
Effective implementation of hyperlocal air quality monitoring involves careful planning and ongoing management:
- Strategic Sensor Placement: Simply deploying sensors randomly is not sufficient. Strategic placement, often guided by initial modeling or analysis of potential pollution sources and population density, is crucial. Sensors should be located near known pollution sources (traffic intersections, industrial areas), in sensitive locations (schools, hospitals), and across different urban microenvironments to capture representative data. Mobile monitoring using sensors on vehicles (e.g., buses, waste trucks) can also provide valuable spatial coverage.
- Network Design and Scalability: Designing a network architecture that is both robust and scalable is essential. This involves selecting appropriate communication protocols, ensuring sufficient network coverage, and planning for the ability to easily add more sensors as needed to expand coverage or increase density.
- Data Governance and Management: Managing the large volume of data from a hyperlocal network requires clear data governance policies. This includes defining data ownership, establishing data storage protocols, ensuring data security and privacy, and determining how data will be accessed and shared among different city departments, researchers, and the public.
- Integration with Existing Infrastructure: Hyperlocal networks should ideally complement and integrate with existing regulatory monitoring stations, using the high-accuracy data from regulatory sites for calibration and validation of the LCS network. Integration with other smart city platforms, such as traffic management or weather systems, enhances the analytical capabilities and potential for targeted interventions.
The Benefits of Granular Air Quality Data
The power of hyperlocal monitoring lies in the granular data it provides, offering numerous benefits for urban air quality management:
- Pinpointing Localized Pollution Sources and Hotspots: Granular data allows cities to move beyond identifying general polluted areas to pinpointing specific streets, intersections, or even buildings that are significant contributors to air pollution. This precision is essential for implementing targeted emission controls or regulatory enforcement.
- Improved Understanding of Human Exposure: Hyperlocal data provides a much more accurate representation of the air quality individuals are exposed to as they move through different parts of the city throughout the day, offering valuable insights for public health assessments and personal health decisions.
- Informing Targeted Interventions and Policies: With detailed data, cities can design and implement highly specific and effective pollution reduction measures. This could include implementing localized traffic calming or rerouting during peak pollution times, targeting emissions from specific industrial facilities, or deploying green infrastructure in areas shown to have the greatest need.
- Empowering Citizens with Local Information: Providing residents with real-time, hyperlocal air quality data directly impacts their lives. It allows them to make informed decisions about their daily routines, such as choosing less polluted routes for exercise or limiting outdoor exposure on high-pollution days. This transparency also builds trust and can encourage citizen participation in air quality improvement efforts.
- Supporting Urban Planning and Development Decisions: Granular air quality data can inform urban planning decisions, such as the location of new schools, hospitals, or residential areas, and guide the design of urban infrastructure to minimize pollution exposure in sensitive zones.
- Enabling More Accurate Predictive Modeling: Higher spatial and temporal resolution data from hyperlocal networks provides more robust inputs for air quality forecasting models, improving their accuracy and ability to predict pollution events.
Challenges in Implementing Hyperlocal Monitoring
Despite the significant benefits, deploying and managing hyperlocal air quality networks comes with challenges:
- Ensuring Data Accuracy and Reliability: As noted earlier, maintaining the accuracy and reliability of data from low-cost sensors over time and in varying environmental conditions is a significant challenge. This requires robust calibration, validation, and ongoing quality control procedures.
- Data Management, Storage, and Processing at Scale: The sheer volume of data generated by a dense network can be immense, creating technical and financial challenges related to data storage, processing, and analysis infrastructure.
- Calibration and Maintenance Logistics: While individual sensors are low-cost, the logistics and resources required to regularly calibrate, maintain, and potentially replace a large number of distributed sensors across a city can be substantial.
- Cybersecurity Risks for Distributed Networks: A large network of interconnected IoT devices represents a potential attack surface for cyber threats. Ensuring the security of the sensors, communication network, and data platform is critical to prevent data tampering or system disruption.
- Integrating Data from Heterogeneous Sensor Types: Hyperlocal networks may utilize sensors from different manufacturers with varying data formats and characteristics. Integrating and standardizing this heterogeneous data for centralized analysis can be complex.
- Translating Complex Data into Actionable Insights for Non-Experts: Granular data can be complex. Presenting this data and its implications in a clear, understandable, and actionable way for policymakers, urban planners, and the general public requires effective visualization and communication tools.
Smart City SS and Hyperlocal Air Quality Monitoring Solutions
Smart City Strategies & Solutions (Smart City SS) is at the forefront of providing comprehensive solutions for hyperlocal air quality monitoring in urban environments. We understand the power of granular data in combating air pollution and improving public health. Our expertise includes designing and deploying scalable IoT sensor networks tailored to the specific needs and geography of a city, implementing robust data platforms for the ingestion, storage, and analysis of high-resolution air quality data, and providing advanced analytics and visualization tools that translate complex data into actionable insights. We also offer services related to sensor calibration, data validation, and integration with existing smart city infrastructure. By partnering with Smart City SS, cities can effectively leverage the power of hyperlocal monitoring to gain a deeper understanding of their air quality, implement targeted pollution reduction strategies, and create healthier, more informed communities.
The Future of Hyperlocal Air Quality Data
The field of hyperlocal air quality monitoring is continuously evolving. We can anticipate further miniaturization of sensors, making them even easier to deploy and potentially integrate into mobile phones or wearable devices. Advanced AI algorithms will enable more sophisticated real-time source apportionment, identifying the specific types and locations of emissions contributing to pollution in a given area. The integration of hyperlocal data with other datasets, such as meteorological data, traffic patterns, and even social media, will provide even richer insights. Furthermore, citizen science initiatives, empowered by accessible low-cost sensors and data platforms, are likely to play a growing role in expanding monitoring coverage and increasing public engagement.
Conclusion: Seeing the Air We Breathe – Granular Data for Healthier Cities
Hyperlocal air quality monitoring, enabled by the deployment of dense IoT sensor networks, represents a transformative leap forward in combating urban air pollution. By moving beyond broad averages to capture granular, real-time data, cities can gain an unprecedented understanding of pollution hotspots, sources, and human exposure. This detailed information is essential for developing and implementing targeted, effective pollution reduction strategies that protect public health and enhance environmental quality. While challenges related to data accuracy, management, and integration exist, the benefits of seeing the air we breathe with such clarity are undeniable. Smart City SS is dedicated to providing the innovative solutions and expertise that cities need to harness the power of hyperlocal monitoring and build cleaner, healthier urban futures. Contact Smart City SS today to learn how our solutions can help you implement a powerful hyperlocal air quality monitoring system in your city.
